次へ: Another Interpretation as Clustering
上へ: A Maximum Likelihood Formulation
戻る: Model of Harmonic Structures
Model Parameter Estimation using EM Algorithm
Since the observed spectral density function
, where
denotes
log-frequency, is considered to be
generated from the model of multiple harmonic structures, the log-likelihood difference
in accordance with an update of the model
parameter
to
is
 |
|
|
(2) |
Although Dempster formulated EM algorithm [8] in order to maximize the mean
log-likelihood considering
as a probabilistic density function,
it can also be formulated in a same way even if
is replaced with
spectral density function.
By taking expectation of both sides with respect to
which represents the probability of the
-labeled Gaussian
distribution from which
is generated,
-function will be derived
in the right-hand side.
Given
-function as
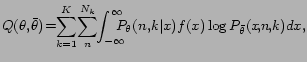 |
|
|
(3) |
thus it yields
 |
|
|
(4) |
By obtaining
which maximizes the
function, the log-likelihood of the model of multiple harmonic structures with
respect to every
will be monotonously increased. A posteriori
probability
in equation (3) is given as
where
is a Gaussian distribution.
By the iterative procedure of the two steps as follows,
the model parameter
locally converges to ML estimates.
Initial-step
Initialize the model parameter
.
Expectaion-step
Calculate
with equation (3).
Maximization-step
Maximize
to obtain the next estimate
 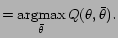 |
|
|
(8) |
Replace
with
and repeat from the Expectation-step.
次へ: Another Interpretation as Clustering
上へ: A Maximum Likelihood Formulation
戻る: Model of Harmonic Structures
平成16年3月25日